2021/04/18
機械学習もできるデスクトップパソコンを自作しました
ubuntutensorflowmachine-learning
やったこと
RTX3090を搭載した機械学習もできそうなデスクトップパソコンを自作しました!
ついでに、tensorflowの最新コードをビルドして、tensorflowのベンチマーク的なものを計測しました。
経緯
- 半年前(2020年秋)に自作のPCパーツをかいました。
- 半年前はRTX3080をとある電気量販店で購入しましたが・・半年経っても一向に届く見込みがありませんでした。(GPUの需要高騰と大量生産されない製品などで、転売されやすい背景が原因なんでしょうかね・・・)
- 年末から今までにかけて仕事がそこそこ忙しく、ずっと放置してたのですが・・さすがに眠っているパーツ達に申し訳がなかったので、半分ヤケになって倍以上はするであろうRTX3090を購入して組立を決意しました!
作業内容
以下に作業した内容を記録しておきます。
パーツの選定・購入
以下を選定しました。
種類 | 名前 | 単価 | 購入数 | 合計金額 |
---|---|---|---|---|
OS | Ubuntu 20.04 LTS | 0円 | 1 | 0円 |
CPU | Ryzen 9 3900X BOX | 57,980円 | 1個 | 57,980円 |
CPUクーラー | 虎徹 MarkII SCKTT-2000 | 4,000円 | 1個 | 4,000円 |
CPUグリス | アイネックス シルバーグリス AK-450-SS | 500円 | 1個 | 500円 |
メモリ | DDR4-2666 DIMM (PC4-21300) 16GB | 8,118円 | 4枚 | 32,472円 |
SSD | NVMe対応 M.2 SSD 1TB | 12,799円 | 4枚 | 12,799円 |
GPU | ASUS GEFORCE RTX3090 24GB | 300,000円 | 1枚 | 300,000円 |
マザーボード | MEG X570 UNIFY | 26,500円 | 1枚 | 26,500円 |
PCケース | bequiet BGW28 | 28,090円 | 1個 | 28,090円 |
電源 | SF750 Platinum CP-9020186-JP | 19,679円 | 1個 | 19,679円 |
電源ケーブル | CableMod Classic ModMesh C-Series Cable Kit for Corsair RMi & RMx | 7,000円 | 1個 | 7,000円 |
合計金額 | 489,020円 |
以下注意点です。
- 電源の選定は完全にミスでした。
- ATX対応はしているんですが、PCケースとの相性がよくありませんでした。
- 付属しているケーブルが短く(多分Micro ATX用にコンパクトに作られているから?)私が購入した大きめのPCケースだと取り付ける電源の位置の関係で、配線が物理的にできない問題が発生しました
- なので、追加で電源ケーブルを購入する羽目になりました。
- 割と追加で買った電源ケーブル(CableMod Classic ModMesh C-Series Cable Kit for Corsair RMi & RMx)がおしゃれだったので、心の落とし所はつけれたんですが・・電源ケーブル追加費用は痛いところ
- 家にマウスやキーボードは余っていたものがあったので購入していません。
- GPU/CPUグリス/CPUクーラーの値段はうる覚え(
購入履歴を調べるのが面倒)なので、市場価格を載せています - 他にも共同でこのマシンを使う人用に以下も購入したり、ありものを使っています
- crucialの500GBのSSD
- windows 10 Pro 64GBのライセンス
届いたパーツ達
cpu
cpuクーラー
cpuグリス
gpu
マザーボード
メモリ
SSD
!
電源
電源ケーブル
PCケース
組立
以下の順に組立ます。
CPUの取り付け
CPUを取り出しマザーボードに慎重に取り付けます。
- 方向を間違えるとCPUのピンを傷つけてしまうので、注意しながら作業します
- 曲がりやすく慎重に作業する必要があるのですが、蓋をするときは結構力がいるという怖い作業です
CPUがマザーボードに付けれたら、CPUクーラーをつけるための土台を取り付けます。
土台をつけることができたら、CPUグリスをCPUの上に(結構雑に)塗り、CPUクーラーを取り付けます。
- 手に擦り傷がいっぱいできます。(薄いゴム手袋のようなもので保護した方が良いかも)
- CPUクーラーがちゃんと回るように配線します。
メモリの取り付け
CPUクーラーが取り付けられたら、メモリーを指定の箇所に差し込みます。
- 結構力がいるので、こちらも注意が必要です。
SSD取り付け
次にM.2という種類のSSD(1TB)を取り付けます。
- 最近(M.2)のはCPUの付近に取り付けれて、カードタイプでペラペラなのに1TBもあります(スゴイ)。
- マザーボードのM.2 SSD用のカバーを取り外して付けてあげます。
- SSDを保護するためのカバーを取り付けてあげます。
M.2タイプのSSDの取り付けが終わったら、サブのSSD500GBをケースの裏側に取り付けます。
GPUの取り付け
最後にGPUを差し込んでパーツの取り付けは完了です。
電源の取り付け
次に電源ケーブルの配線をします。
・・・😅😅😅😅・・ケーブルが短く、電源をPCケースの指定された位置に置けない事態が発生・・・
追加で電源ケーブルを購入する羽目になりました。
以下、追加で購入したケーブルで、(ちゃんと)配線できた様子です。
- ケーブルを束ねるプラスチック製のアレ(結束バンド?)が地味に活躍します。
あとは電源をつなぎ、モニター・マウス・キーボードなどを繋げて完成です。
完成図
正面
- 後述しますが、SSD500GBをSATA1に付けていると、M.2のSSDをブートディスクとして認識してくれないので、SSD500GBを一時的に取り外して作業しています😭
背面
OS(Ubuntu)のインストール
この記事で記載した起動用USBを利用します。
OSインストールに際して以下かなり参考になったので、記載しておきます。
- Ubuntu 18.04 その96 - Ubuntuやフレーバーインストール時、インストーラーの画面がディスプレイからはみ出す場合は - kledgeb
- Ubuntu 18.04 インストール(UEFI) その2 - UEFIのPCにUbuntu 18.04をインストールする(パーティションの作成 〜 ブートローダーの設定) - kledgeb
- M.2 SSD がBIOSの起動優先順位に表示されないときの対処法。 | ねんごたれログ
- Ubuntu20.04のMozcでかな切り替えをCtrl+Spaceにする · kapieciiのブログ
- Mozc でキーボードの配列が日本語にならないのを解決する - Qiita
tensorflowの実行環境準備
cuda toolkit / nvidiaドライバーをインストールする
- cuda toolkitをインストールすると対応するnvidiaドライバーもインストールされる模様
$ wget https://developer.download.nvidia.com/compute/cuda/repos/ubuntu2004/x86_64/cuda-ubuntu2004.pin
$ sudo mv cuda-ubuntu2004.pin /etc/apt/preferences.d/cuda-repository-pin-600
$ sudo apt-key adv --fetch-keys https://developer.download.nvidia.com/compute/cuda/repos/ubuntu2004/x86_64/7fa2af80.pub
$ sudo add-apt-repository "deb https://developer.download.nvidia.com/compute/cuda/repos/ubuntu2004/x86_64/ /"
$ sudo apt-get update
$ sudo apt-get -y install cuda-11-2
とりあえず再起動
$ sudo reboot
$ vim ~/.bashrc
CUDA_VERSION=11.2
export PATH=${PATH}:/usr/local/cuda-${CUDA_VERSION}/bin
export LD_LIBRARY_PATH=${LD_LIBRARY_PATH:+${LD_LIBRARY_PATH}:}/usr/local/cuda-${CUDA_VERSION}/lib64
$ source ~/.bashrc
$ sudo ldconfig
$ nvcc -V
nvcc: NVIDIA (R) Cuda compiler driver
Copyright (c) 2005-2021 NVIDIA Corporation
Built on Sun_Feb_14_21:12:58_PST_2021
Cuda compilation tools, release 11.2, V11.2.152
Build cuda_11.2.r11.2/compiler.29618528_0
$ nvidia-smi
Sat Apr 17 07:42:09 2021
+-----------------------------------------------------------------------------+
| NVIDIA-SMI 465.19.01 Driver Version: 465.19.01 CUDA Version: 11.3 |
|-------------------------------+----------------------+----------------------+
| GPU Name Persistence-M| Bus-Id Disp.A | Volatile Uncorr. ECC |
| Fan Temp Perf Pwr:Usage/Cap| Memory-Usage | GPU-Util Compute M. |
| | | MIG M. |
|===============================+======================+======================|
| 0 NVIDIA GeForce ... On | 00000000:2D:00.0 On | N/A |
| 0% 39C P8 18W / 350W | 270MiB / 24260MiB | 1% Default |
| | | N/A |
+-------------------------------+----------------------+----------------------+
+-----------------------------------------------------------------------------+
| Processes: |
| GPU GI CI PID Type Process name GPU Memory |
| ID ID Usage |
|=============================================================================|
| 0 N/A N/A 1018 G /usr/lib/xorg/Xorg 35MiB |
| 0 N/A N/A 1546 G /usr/lib/xorg/Xorg 105MiB |
| 0 N/A N/A 1676 G /usr/bin/gnome-shell 74MiB |
| 0 N/A N/A 3784 G /usr/lib/firefox/firefox 4MiB |
| 0 N/A N/A 4275 G ...AAAAAAAA== --shared-files 34MiB |
+-----------------------------------------------------------------------------+
dockerをインストール
tensorflowのdockerコンテナ内での実行が便利なようなので、ひとまずdockerを準備します。
$ sudo apt update
$ sudo apt install apt-transport-https ca-certificates curl software-properties-common
$ curl -fsSL https://download.docker.com/linux/ubuntu/gpg | sudo apt-key add -
$ sudo add-apt-repository "deb [arch=amd64] https://download.docker.com/linux/ubuntu focal stable"
$ sudo apt update
$ sudo apt install docker-ce
# dockerをログインユーザーから使えるようにする
$ sudo usermod -aG docker ${USER}
# ログインし直すことで、docker infoなどが見れるようになる
nvidia-dockerのインストール
Installation Guide — NVIDIA Cloud Native Technologies documentation
$ distribution=$(. /etc/os-release;echo $ID$VERSION_ID) \
&& curl -s -L https://nvidia.github.io/nvidia-docker/gpgkey | sudo apt-key add - \
&& curl -s -L https://nvidia.github.io/nvidia-docker/$distribution/nvidia-docker.list | sudo tee /etc/apt/sources.list.d/nvidia-docker.list
$ curl -s -L https://nvidia.github.io/nvidia-container-runtime/experimental/$distribution/nvidia-container-runtime.list | sudo tee /etc/apt/sources.list.d/nvidia-container-runtime.list
$ sudo apt-get update
$ sudo apt-get install -y nvidia-docker2
$ sudo systemctl restart docker
tensorflowのビルド
$ docker pull tensorflow/tensorflow:devel-gpu
$ docker run --gpus all -it -w /tensorflow -v $PWD:/mnt -e HOST_PERMS="$(id -u):$(id -g)" \
tensorflow/tensorflow:devel-gpu bash
# cd /tensorflow_src
# git pull
# ./configure
# bazel build --config=opt --config=cuda //tensorflow/tools/pip_package:build_pip_package
# ./bazel-bin/tensorflow/tools/pip_package/build_pip_package /mnt
# chown $HOST_PERMS /mnt/tensorflow-2.6.0-cp36-cp36m-linux_x86_64.whl
# pip install /mnt/tensorflow-2.6.0-cp36-cp36m-linux_x86_64.whl
# pip show tensorflow
Name: tensorflow
Version: 2.6.0
Summary: TensorFlow is an open source machine learning framework for everyone.
Home-page: https://www.tensorflow.org/
Author: Google Inc.
Author-email: [email protected]
License: Apache 2.0
Location: /usr/local/lib/python3.6/dist-packages
Requires: flatbuffers, gast, google-pasta, tensorflow-estimator, absl-py, termcolor, keras-preprocessing, opt-einsum, h5py, six, wheel, tensorboard, protobuf, grpcio, numpy, wrapt, typing-extensions, keras-nightly, astunparse
Required-by:
# cd /tmp
# python
- ビルドできたので、以下動作確認
>>> import tensorflow as tf
2021-04-17 05:58:51.740733: I tensorflow/stream_executor/platform/default/dso_loader.cc:53] Successfully opened dynamic library libcudart.so.11.0
INFO:tensorflow:Enabling eager execution
INFO:tensorflow:Enabling v2 tensorshape
INFO:tensorflow:Enabling resource variables
INFO:tensorflow:Enabling tensor equality
INFO:tensorflow:Enabling control flow v2
>>> print("Num GPUs Available: ", len(tf.config.list_physical_devices('GPU')))
2021-04-17 06:00:41.261039: I tensorflow/stream_executor/platform/default/dso_loader.cc:53] Successfully opened dynamic library libcuda.so.1
2021-04-17 06:00:41.297013: I tensorflow/stream_executor/cuda/cuda_gpu_executor.cc:937] successful NUMA node read from SysFS had negative value (-1), but there must be at least one NUMA node, so returning NUMA node zero
2021-04-17 06:00:41.297691: I tensorflow/core/common_runtime/gpu/gpu_device.cc:1734] Found device 0 with properties:
pciBusID: 0000:2d:00.0 name: NVIDIA GeForce RTX 3090 computeCapability: 8.6
coreClock: 1.74GHz coreCount: 82 deviceMemorySize: 23.69GiB deviceMemoryBandwidth: 871.81GiB/s
2021-04-17 06:00:41.297708: I tensorflow/stream_executor/platform/default/dso_loader.cc:53] Successfully opened dynamic library libcudart.so.11.0
2021-04-17 06:00:41.299516: I tensorflow/stream_executor/platform/default/dso_loader.cc:53] Successfully opened dynamic library libcublas.so.11
2021-04-17 06:00:41.299541: I tensorflow/stream_executor/platform/default/dso_loader.cc:53] Successfully opened dynamic library libcublasLt.so.11
2021-04-17 06:00:41.300119: I tensorflow/stream_executor/platform/default/dso_loader.cc:53] Successfully opened dynamic library libcufft.so.10
2021-04-17 06:00:41.300259: I tensorflow/stream_executor/platform/default/dso_loader.cc:53] Successfully opened dynamic library libcurand.so.10
2021-04-17 06:00:41.300789: I tensorflow/stream_executor/platform/default/dso_loader.cc:53] Successfully opened dynamic library libcusolver.so.11
2021-04-17 06:00:41.301235: I tensorflow/stream_executor/platform/default/dso_loader.cc:53] Successfully opened dynamic library libcusparse.so.11
2021-04-17 06:00:41.301312: I tensorflow/stream_executor/platform/default/dso_loader.cc:53] Successfully opened dynamic library libcudnn.so.8
2021-04-17 06:00:41.301385: I tensorflow/stream_executor/cuda/cuda_gpu_executor.cc:937] successful NUMA node read from SysFS had negative value (-1), but there must be at least one NUMA node, so returning NUMA node zero
2021-04-17 06:00:41.302064: I tensorflow/stream_executor/cuda/cuda_gpu_executor.cc:937] successful NUMA node read from SysFS had negative value (-1), but there must be at least one NUMA node, so returning NUMA node zero
2021-04-17 06:00:41.302727: I tensorflow/core/common_runtime/gpu/gpu_device.cc:1872] Adding visible gpu devices: 0
Num GPUs Available: 1
tensorflow benchmarkの実行
tensorflowのビルドを実行したdockerコンテナ内で、github.com/tensorflow/benchmarksをダウンロードして、ベンチマークのスクリプトを実行しました。
# cd /tmp
# git clone https://github.com/tensorflow/benchmarks
# cd ./benchmarks/scripts/tf_cnn_benchmarks/
# python tf_cnn_benchmarks.py --num_gpus=1 --batch_size=32 --model=resnet50 --variable_update=parameter_server
2021-04-17 06:09:18.225635: I tensorflow/stream_executor/platform/default/dso_loader.cc:53] Successfully opened dynamic library libcudart.so.11.0
WARNING:tensorflow:From /usr/local/lib/python3.6/dist-packages/tensorflow/python/compat/v2_compat.py:96: disable_resource_variables (from tensorflow.python.ops.variable_scope) is deprecated and will be removed in a future version.
Instructions for updating:
non-resource variables are not supported in the long term
2021-04-17 06:09:19.082986: I tensorflow/core/platform/cpu_feature_guard.cc:142] This TensorFlow binary is optimized with oneAPI Deep Neural Network Library (oneDNN) to use the following CPU instructions in performance-critical operations: SSE3 SSE4.1 SSE4.2 AVX AVX2 FMA
To enable them in other operations, rebuild TensorFlow with the appropriate compiler flags.
2021-04-17 06:09:19.083613: I tensorflow/stream_executor/platform/default/dso_loader.cc:53] Successfully opened dynamic library libcuda.so.1
2021-04-17 06:09:19.117190: I tensorflow/stream_executor/cuda/cuda_gpu_executor.cc:937] successful NUMA node read from SysFS had negative value (-1), but there must be at least one NUMA node, so returning NUMA node zero
2021-04-17 06:09:19.118308: I tensorflow/core/common_runtime/gpu/gpu_device.cc:1734] Found device 0 with properties:
pciBusID: 0000:2d:00.0 name: NVIDIA GeForce RTX 3090 computeCapability: 8.6
coreClock: 1.74GHz coreCount: 82 deviceMemorySize: 23.69GiB deviceMemoryBandwidth: 871.81GiB/s
2021-04-17 06:09:19.118336: I tensorflow/stream_executor/platform/default/dso_loader.cc:53] Successfully opened dynamic library libcudart.so.11.0
2021-04-17 06:09:19.120404: I tensorflow/stream_executor/platform/default/dso_loader.cc:53] Successfully opened dynamic library libcublas.so.11
2021-04-17 06:09:19.120434: I tensorflow/stream_executor/platform/default/dso_loader.cc:53] Successfully opened dynamic library libcublasLt.so.11
2021-04-17 06:09:19.121028: I tensorflow/stream_executor/platform/default/dso_loader.cc:53] Successfully opened dynamic library libcufft.so.10
2021-04-17 06:09:19.121169: I tensorflow/stream_executor/platform/default/dso_loader.cc:53] Successfully opened dynamic library libcurand.so.10
2021-04-17 06:09:19.121707: I tensorflow/stream_executor/platform/default/dso_loader.cc:53] Successfully opened dynamic library libcusolver.so.11
2021-04-17 06:09:19.122151: I tensorflow/stream_executor/platform/default/dso_loader.cc:53] Successfully opened dynamic library libcusparse.so.11
2021-04-17 06:09:19.122230: I tensorflow/stream_executor/platform/default/dso_loader.cc:53] Successfully opened dynamic library libcudnn.so.8
2021-04-17 06:09:19.122306: I tensorflow/stream_executor/cuda/cuda_gpu_executor.cc:937] successful NUMA node read from SysFS had negative value (-1), but there must be at least one NUMA node, so returning NUMA node zero
2021-04-17 06:09:19.123007: I tensorflow/stream_executor/cuda/cuda_gpu_executor.cc:937] successful NUMA node read from SysFS had negative value (-1), but there must be at least one NUMA node, so returning NUMA node zero
2021-04-17 06:09:19.123655: I tensorflow/core/common_runtime/gpu/gpu_device.cc:1872] Adding visible gpu devices: 0
2021-04-17 06:09:19.123677: I tensorflow/stream_executor/platform/default/dso_loader.cc:53] Successfully opened dynamic library libcudart.so.11.0
2021-04-17 06:15:26.911296: I tensorflow/core/common_runtime/gpu/gpu_device.cc:1258] Device interconnect StreamExecutor with strength 1 edge matrix:
2021-04-17 06:15:26.911322: I tensorflow/core/common_runtime/gpu/gpu_device.cc:1264] 0
2021-04-17 06:15:26.911326: I tensorflow/core/common_runtime/gpu/gpu_device.cc:1277] 0: N
2021-04-17 06:15:26.911506: I tensorflow/stream_executor/cuda/cuda_gpu_executor.cc:937] successful NUMA node read from SysFS had negative value (-1), but there must be at least one NUMA node, so returning NUMA node zero
2021-04-17 06:15:26.912172: I tensorflow/stream_executor/cuda/cuda_gpu_executor.cc:937] successful NUMA node read from SysFS had negative value (-1), but there must be at least one NUMA node, so returning NUMA node zero
2021-04-17 06:15:26.912809: I tensorflow/stream_executor/cuda/cuda_gpu_executor.cc:937] successful NUMA node read from SysFS had negative value (-1), but there must be at least one NUMA node, so returning NUMA node zero
2021-04-17 06:15:26.913432: I tensorflow/core/common_runtime/gpu/gpu_device.cc:1418] Created TensorFlow device (/job:localhost/replica:0/task:0/device:GPU:0 with 22149 MB memory) -> physical GPU (device: 0, name: NVIDIA GeForce RTX 3090, pci bus id: 0000:2d:00.0, compute capability: 8.6)
TensorFlow: 2.6
Model: resnet50
Dataset: imagenet (synthetic)
Mode: training
SingleSess: False
Batch size: 32 global
32 per device
Num batches: 100
Num epochs: 0.00
Devices: ['/gpu:0']
NUMA bind: False
Data format: NCHW
Optimizer: sgd
Variables: parameter_server
==========
Generating training model
/usr/local/lib/python3.6/dist-packages/tensorflow/python/keras/legacy_tf_layers/convolutional.py:414: UserWarning: `tf.layers.conv2d` is deprecated and will be removed in a future version. Please Use `tf.keras.layers.Conv2D` instead.
warnings.warn('`tf.layers.conv2d` is deprecated and '
/usr/local/lib/python3.6/dist-packages/tensorflow/python/keras/engine/base_layer_v1.py:1694: UserWarning: `layer.apply` is deprecated and will be removed in a future version. Please use `layer.__call__` method instead.
warnings.warn('`layer.apply` is deprecated and '
WARNING:tensorflow:From /usr/local/lib/python3.6/dist-packages/tensorflow/python/keras/layers/normalization.py:533: _colocate_with (from tensorflow.python.framework.ops) is deprecated and will be removed in a future version.
Instructions for updating:
Colocations handled automatically by placer.
W0417 06:15:26.940969 140092129843008 deprecation.py:336] From /usr/local/lib/python3.6/dist-packages/tensorflow/python/keras/layers/normalization.py:533: _colocate_with (from tensorflow.python.framework.ops) is deprecated and will be removed in a future version.
Instructions for updating:
Colocations handled automatically by placer.
/usr/local/lib/python3.6/dist-packages/tensorflow/python/keras/legacy_tf_layers/pooling.py:310: UserWarning: `tf.layers.max_pooling2d` is deprecated and will be removed in a future version. Please use `tf.keras.layers.MaxPooling2D` instead.
warnings.warn('`tf.layers.max_pooling2d` is deprecated and '
Initializing graph
WARNING:tensorflow:From /tmp/benchmarks/scripts/tf_cnn_benchmarks/benchmark_cnn.py:2268: Supervisor.__init__ (from tensorflow.python.training.supervisor) is deprecated and will be removed in a future version.
Instructions for updating:
Please switch to tf.train.MonitoredTrainingSession
W0417 06:15:28.324391 140092129843008 deprecation.py:336] From /tmp/benchmarks/scripts/tf_cnn_benchmarks/benchmark_cnn.py:2268: Supervisor.__init__ (from tensorflow.python.training.supervisor) is deprecated and will be removed in a future version.
Instructions for updating:
Please switch to tf.train.MonitoredTrainingSession
2021-04-17 06:15:28.512207: I tensorflow/stream_executor/cuda/cuda_gpu_executor.cc:937] successful NUMA node read from SysFS had negative value (-1), but there must be at least one NUMA node, so returning NUMA node zero
2021-04-17 06:15:28.512920: I tensorflow/core/common_runtime/gpu/gpu_device.cc:1734] Found device 0 with properties:
pciBusID: 0000:2d:00.0 name: NVIDIA GeForce RTX 3090 computeCapability: 8.6
coreClock: 1.74GHz coreCount: 82 deviceMemorySize: 23.69GiB deviceMemoryBandwidth: 871.81GiB/s
2021-04-17 06:15:28.513136: I tensorflow/stream_executor/cuda/cuda_gpu_executor.cc:937] successful NUMA node read from SysFS had negative value (-1), but there must be at least one NUMA node, so returning NUMA node zero
2021-04-17 06:15:28.513775: I tensorflow/stream_executor/cuda/cuda_gpu_executor.cc:937] successful NUMA node read from SysFS had negative value (-1), but there must be at least one NUMA node, so returning NUMA node zero
2021-04-17 06:15:28.514371: I tensorflow/core/common_runtime/gpu/gpu_device.cc:1872] Adding visible gpu devices: 0
2021-04-17 06:15:28.514398: I tensorflow/core/common_runtime/gpu/gpu_device.cc:1258] Device interconnect StreamExecutor with strength 1 edge matrix:
2021-04-17 06:15:28.514405: I tensorflow/core/common_runtime/gpu/gpu_device.cc:1264] 0
2021-04-17 06:15:28.514409: I tensorflow/core/common_runtime/gpu/gpu_device.cc:1277] 0: N
2021-04-17 06:15:28.514469: I tensorflow/stream_executor/cuda/cuda_gpu_executor.cc:937] successful NUMA node read from SysFS had negative value (-1), but there must be at least one NUMA node, so returning NUMA node zero
2021-04-17 06:15:28.515120: I tensorflow/stream_executor/cuda/cuda_gpu_executor.cc:937] successful NUMA node read from SysFS had negative value (-1), but there must be at least one NUMA node, so returning NUMA node zero
2021-04-17 06:15:28.515732: I tensorflow/core/common_runtime/gpu/gpu_device.cc:1418] Created TensorFlow device (/job:localhost/replica:0/task:0/device:GPU:0 with 22149 MB memory) -> physical GPU (device: 0, name: NVIDIA GeForce RTX 3090, pci bus id: 0000:2d:00.0, compute capability: 8.6)
2021-04-17 06:15:28.586725: I tensorflow/core/platform/profile_utils/cpu_utils.cc:114] CPU Frequency: 3800165000 Hz
INFO:tensorflow:Running local_init_op.
I0417 06:15:29.022122 140092129843008 session_manager.py:531] Running local_init_op.
INFO:tensorflow:Done running local_init_op.
I0417 06:15:29.079068 140092129843008 session_manager.py:534] Done running local_init_op.
Running warm up
2021-04-17 06:15:29.861047: I tensorflow/stream_executor/platform/default/dso_loader.cc:53] Successfully opened dynamic library libcudnn.so.8
2021-04-17 06:15:30.459682: I tensorflow/stream_executor/cuda/cuda_dnn.cc:368] Loaded cuDNN version 8100
2021-04-17 06:15:31.342782: I tensorflow/stream_executor/platform/default/dso_loader.cc:53] Successfully opened dynamic library libcublas.so.11
2021-04-17 06:15:31.730796: I tensorflow/stream_executor/platform/default/dso_loader.cc:53] Successfully opened dynamic library libcublasLt.so.11
2021-04-17 06:15:32.111680: I tensorflow/stream_executor/cuda/cuda_blas.cc:1838] TensorFloat-32 will be used for the matrix multiplication. This will only be logged once.
Done warm up
Step Img/sec total_loss
1 images/sec: 418.6 +/- 0.0 (jitter = 0.0) 7.765
10 images/sec: 444.5 +/- 3.6 (jitter = 2.8) 8.049
20 images/sec: 445.8 +/- 2.2 (jitter = 1.9) 7.808
30 images/sec: 446.1 +/- 1.7 (jitter = 2.0) 7.976
40 images/sec: 446.4 +/- 1.4 (jitter = 2.0) 7.591
50 images/sec: 446.6 +/- 1.3 (jitter = 2.1) 7.549
60 images/sec: 446.5 +/- 1.2 (jitter = 2.0) 7.819
70 images/sec: 446.3 +/- 1.2 (jitter = 2.0) 7.819
80 images/sec: 446.4 +/- 1.1 (jitter = 2.1) 7.848
90 images/sec: 446.5 +/- 1.0 (jitter = 2.2) 8.027
100 images/sec: 446.5 +/- 0.9 (jitter = 2.3) 8.032
----------------------------------------------------------------
total images/sec: 446.23
----------------------------------------------------------------
以下ベンチマーク結果です。
total images/sec: 446.23
うーん🤔 多分そこそこ良い感じなんだと思います。(コスパはGPU単体の価格が高いため、そこまでよくなさそう)
以上です!
関連する記事
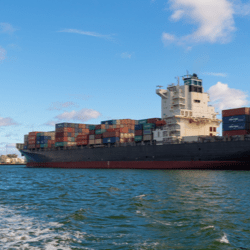
Ubuntu20.04のサーバーにkubeadmを使って開発用のk8sクラスターを作る
シングルサーバー(Ubuntu20.04)構成でオフィスLAN内に開発用のk8sクラスターを立ててみました
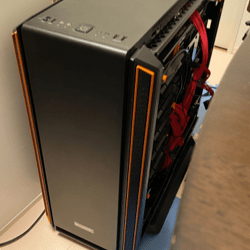
機械学習もできるデスクトップパソコンを自作しました
30万円するGPU(RTX3090)で自作PCを組立て、tensorflowのベンチマークを測ってみました。
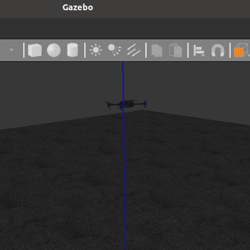
[Ubuntu20.04]Gazebo simulator上のDroneをMAVROSを使って動かす
MAVROSを使ったTutorialを実際に動かしてみました
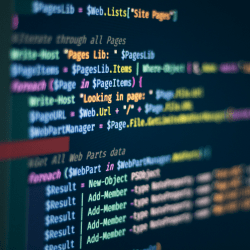
UbuntuにVS Codeをインストールする
Ubuntu20.04にVisual Studio Codeをインストールしてみました